Journal / Conference
Thirty-Fourth AAAI Conference on Artificial Intelligence (AAAI, 2020)
[PDF link: here]
[Code link: here]
Keywords
Weakly-Supervised Learning, Semantic Segmentation
Abstract
Weakly supervised semantic segmentation with only image-level labels saves large human effort to annotate pixel-level labels. Cutting-edge approaches rely on various innovative constraints and heuristic rules to generate the masks for every single image. Although great progress has been achieved by these methods, they treat each image independently and do not take account of the relationships across different images. In this paper, however, we argue that the cross-image relationship is vital for weakly supervised segmentation. Because it connects related regions across images, where supplementary representations can be propagated to obtain more consistent and integral regions. To leverage this information, we propose an end-to-end cross-image affinity module, which exploits pixel-level cross-image relationships with only image-level labels. By means of this, our approach achieves 64.3% and 65.3% mIoU on Pascal VOC 2012 validation and test set respectively, which is a new state-of-the-art result by only using image-level labels for weakly supervised semantic segmentation, demonstrating the superiority of our approach.
Method/Framework
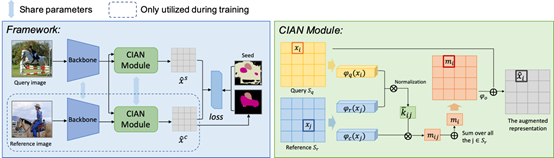
Cross-Image Affinity Net for Weakly Supervised Semantic Segmentation. It learns the cross-image relationships to help weakly-supervised semantic segmentation. The CIAN Module takes features as input from two different images, extract and exchange information across them to augment the original features. The cross-image information is helpful to fully utilize the weak supervision and obtain better predictions.
Experiments
We evaluate the proposed method on the standard Pascal VOC 2012 dataset. Extensive experimental results demonstrate the advantage of utilizing the cross-image relationships.
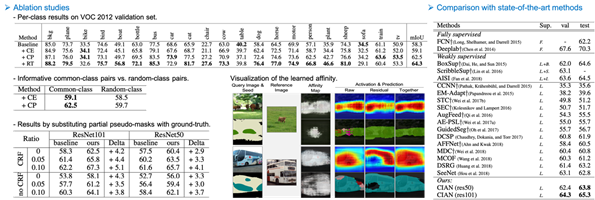
Highlight
• We firstly propose to explicitly model the cross-image relationship for weakly supervised semantic segmentation. An end-to-end cross-image affinity module is proposed to provide supplementary information from related images. By means of this, more integral regions can be obtained for weakly supervised segmentation.
• Extensive experiments demonstrate the usefulness of modeling cross-image relationships. Besides, we show that our approach is orthogonal to the quality of the seeds, which continually improves the training with even better seeds. Thus it can be potentially combined with future works that generate better seeds to further boost the performance.
• With the naive seeds generated by CAM, our CIAN achieves 65.3% mIoU on the VOC 2012 test set, which is a new state-of-the-art result by only using image-level labels for semantic segmentation, demonstrating the superiority of the approach.
Citation
@inproceedings{fan2020cian,
title={CIAN: Cross-Image Affinity Net for Weakly Supervised Semantic Segmentation},
author={Fan, Junsong and Zhang, Zhaoxiang and Tan, Tieniu and Song, Chunfeng and Xiao, Jun},
booktitle={Proceedings of the AAAI Conference on Artificial Intelligence},
year={2020}
}