Journal / Conference
The IEEE International Conference on Computer Vision (ICCV, 2019)
[PDF link:here]
[Code link: here]
Keywords
Video Object Detection, SEquence Level Semantics Aggregation (SELSA)
Abstract
Video objection detection (VID) has been a rising research direction in recent years. A central issue of VID is the appearance degradation of video frames caused by fast motion. This problem is essentially ill-posed for a single frame. Therefore, aggregating features from other frames becomes a natural choice. Existing methods rely heavily on optical flow or recurrent neural networks for feature aggregation. However, these methods emphasize more on the temporally nearby frames. In this work, we argue that aggregating features in the full-sequence level will lead to more discriminative and robust features for video object detection. To achieve this goal, we devise a novel Sequence Level Semantics Aggregation (SELSA) module. We further demonstrate the close relationship between the proposed method and the classic spectral clustering method, providing a novel view for understanding the VID problem. We test the proposed method on the ImageNet VID and the EPIC KITCHENS dataset and achieve new state-of-the-art results. Our method does not need complicated post-processing methods such as Seq-NMS or Tubelet rescoring, which keeps the pipeline simple and clean.
Method/Framework
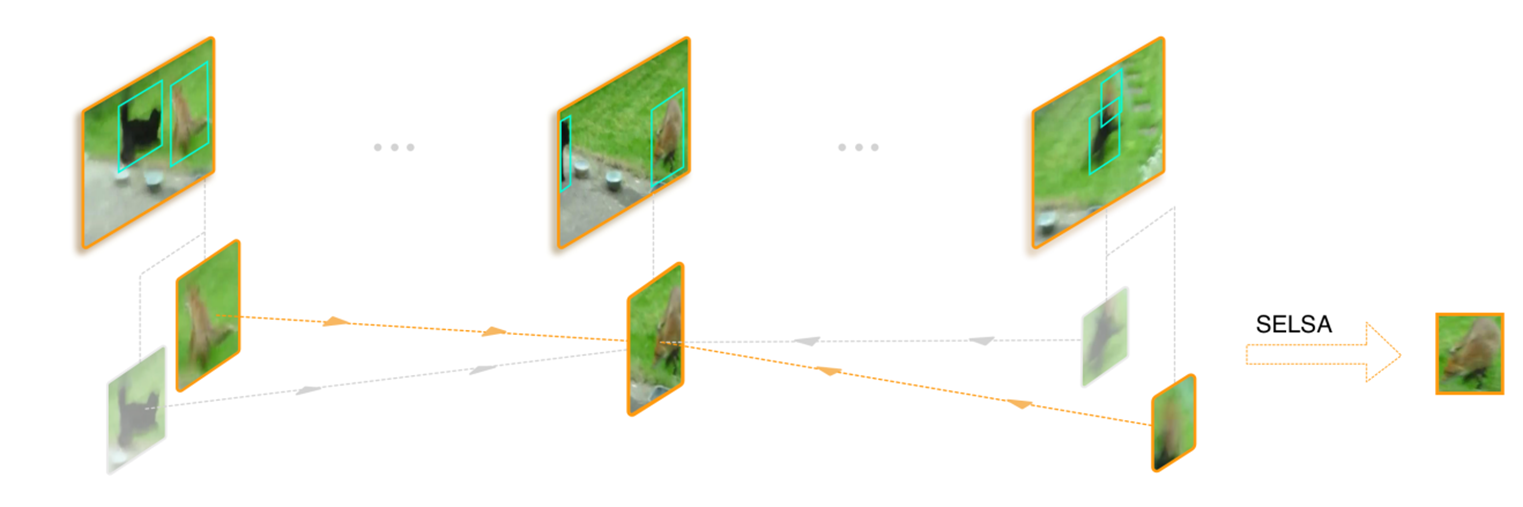
Sequence Level Semantics Aggregation (SELSA) method:The overall architecture of the proposed model. We first extract proposals in different frames from the video, then the semantic similarities of proposals are computed across frames. At last, we aggregate the features from other proposals based on these similarities to obtain a more discriminative and robust features for object detection.
Experiments
We train our model with a mixture of ImageNet VID and DET datasets with the split provided in FGFA. We evaluate our proposed method on ImageNet VID dataset. We report the mAP@IoU=0.5 and motion[1]specific mAP on the validation set.
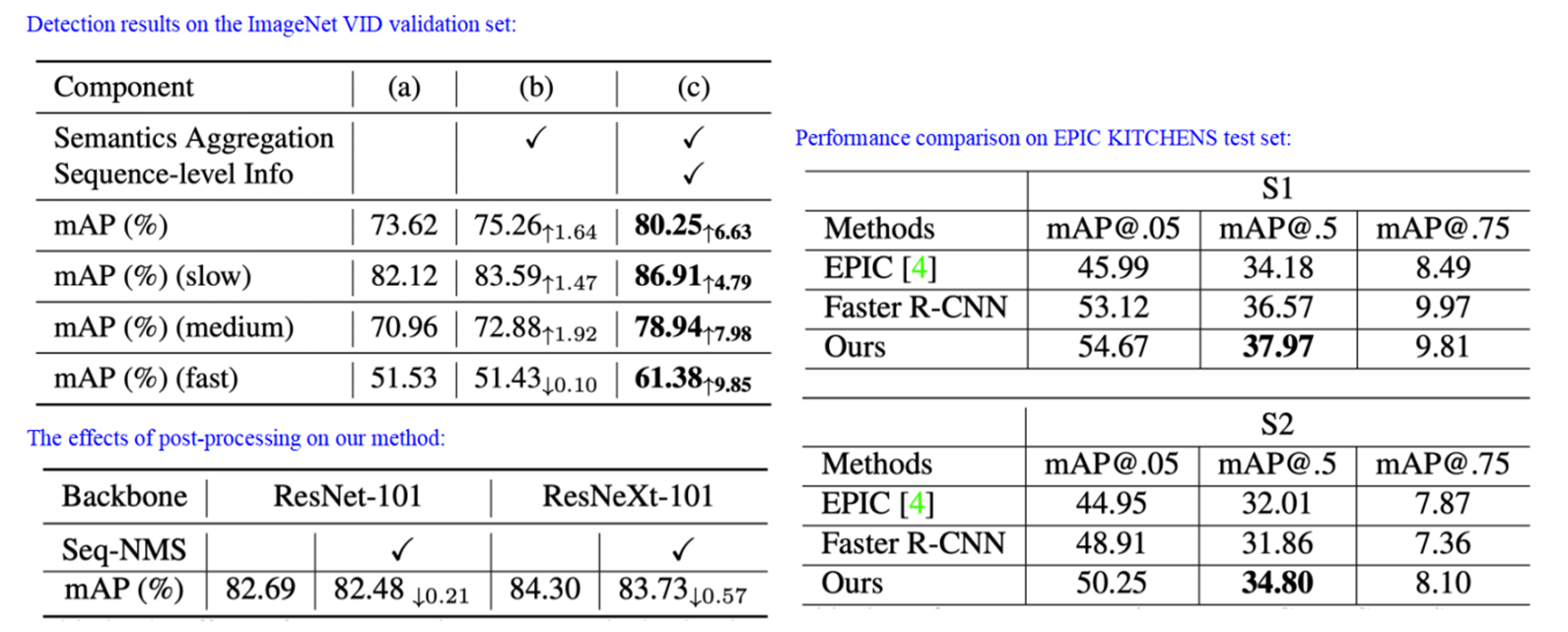
Highlight
- We first treat video detection as a sequence level multi-shot detection problem and then introduce a global clustering viewpoint of VID task for the first time.
- To incorporate such view into current deep object detection pipeline, we introduce a simple but effective Sequence Level Semantics Aggregation (SELSA) module to fully utilize video information.
- We test our proposed method on the large scale ImageNet VID and EPIC KITCHEN datasets and demonstrate significant improvement over previous methods.
- Feature aggregation is essential for video-level tasks.
- Semantics aggregation is simple and effective
Citation
@InProceedings{Wu_2019_ICCV,
author = {Wu, Haiping and Chen, Yuntao and Wang, Naiyan and Zhang, Zhaoxiang},
title = {Sequence Level Semantics Aggregation for Video Object Detection},
booktitle = {The IEEE International Conference on Computer Vision (ICCV)},
month = {October},
year = {2019}}